In today’s financial landscape, the fusion of data and rationality, bolstered by Artificial Intelligence (AI) and Machine Learning (ML), is crucial for informed decision-making and analysis. As data volumes surge, particularly in the digital era, its role in enhancing decision quality is undeniable. AI’s transformative power is revolutionizing financial operations and strategies globally by merging data with rational decision-making.
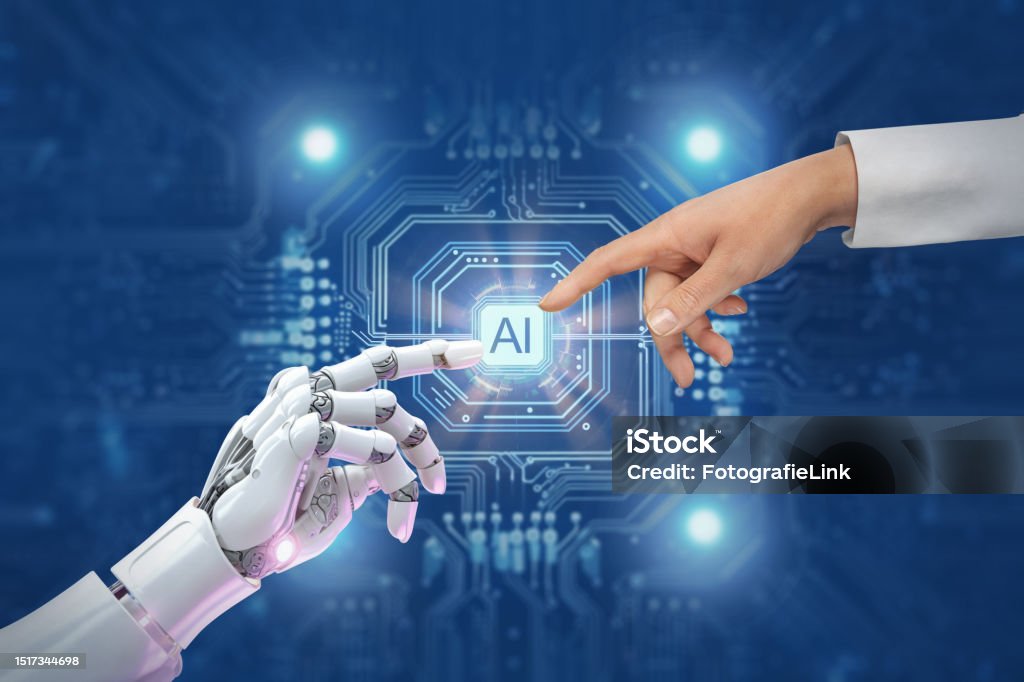
The Core Foundations of AI in Finance
AI’s foundations in finance include:
- Regression Techniques: Enable financial analysts to predict future outcomes based on historical data, improving risk assessment and forecasting accuracy.
- Dimensionality Reduction: Simplifies complex datasets for more manageable analysis.
- Density Estimation: Identifies underlying patterns and distributions in data, aiding in fraud detection and risk management.
- Classification Algorithms: Categorize data into predefined classes, enhancing personalized banking services and targeted marketing strategies.
These pillars support sophisticated AI models crucial for modern financial operations, such as credit card and loan decisions, anti-fraud systems, risk management, stock market prediction, sales forecasting, trading, personalized banking, and process automation.
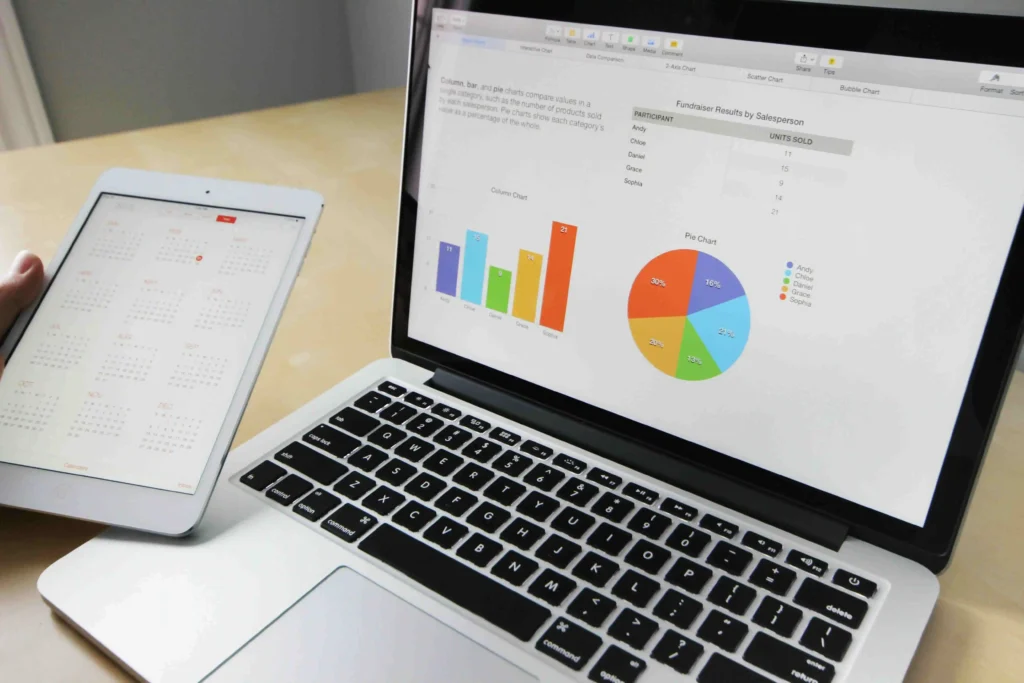
Key Areas of AI Application in Finance
AI has significantly impacted several financial sectors:
- Lending Operations: AI-driven models improve creditworthiness assessment, streamline loan approvals, and mitigate lending risks. Real-time data analysis helps proactively detect potential fraudulent activities, protecting financial institutions and customers.
- Insurance: AI enhances risk assessment and policy personalization by analyzing historical data and customer behavior. AI-powered chatbots and virtual assistants revolutionize customer service, providing prompt and personalized assistance.
- Investment Strategies and Portfolio Management: AI-driven predictive models and algorithmic trading identify lucrative opportunities, optimize portfolios, and mitigate risks. Sales forecasting tools help businesses anticipate market trends, optimize inventory, and improve operational efficiency.
- AI and IoT Synergy: The combination of AI and the Internet of Things (IoT) amplifies data-driven decision-making in finance. IoT devices offer insights into consumer behavior, market trends, and operational efficiency. For example, IoT sensors in retail stores track customer foot traffic, optimize product placement, and personalize marketing campaigns. In banking, IoT devices in ATMs and POS terminals facilitate real-time transaction monitoring and fraud detection.
Challenges and the Way Forward
Despite AI’s benefits in finance, several challenges must be addressed:
- Ethical Use of AI Algorithms: Ensuring unbiased data, especially in credit scoring and loan approval, to prevent discriminatory outcomes.
- Model Complexity and Adversarial Attacks: Protecting AI models from malicious actors manipulating input data.
- Data Privacy and Security: Safeguarding sensitive customer information amidst the proliferation of AI technologies.
Additional challenges include:
- Data Quality and Bias
- Regulatory Compliance
- Cybersecurity Risks
- Ethical Considerations
- Model Interpretability
- Resource Constraints
- Integration with Legacy Systems
- Algorithmic Fairness
- Operational Risks
- Customer Trust and Acceptance
Addressing these challenges requires collaborative efforts from policymakers, regulators, and industry stakeholders to create robust frameworks for responsible AI deployment in finance. Overcoming these obstacles will enable financial institutions to leverage AI’s full potential, driving innovation, enhancing efficiency, and delivering superior value to customers in an increasingly digital world.